Why Financial Firms Struggle with Data Quality and How Automation Brings Consistency
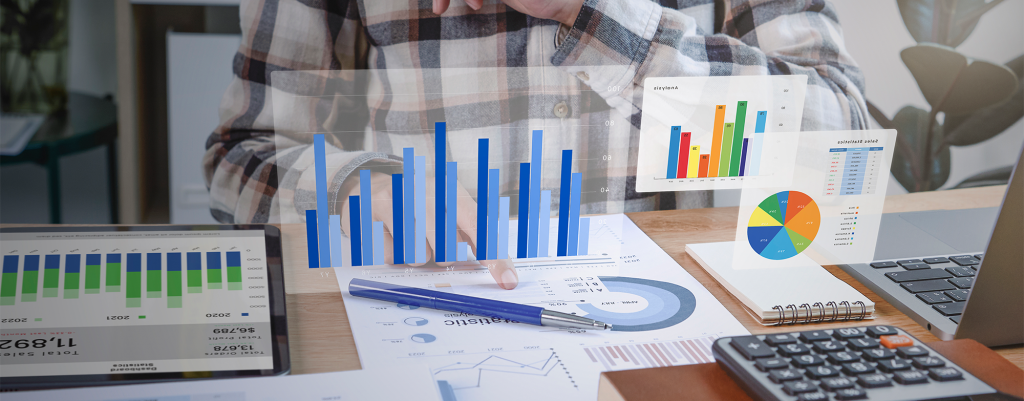
A busy analyst at a financial firm starts her day by preparing reports that are crucial to investment decisions. She pulls data from multiple sources—well-known databases and smaller niche ones. Each dataset has its quirks: differing formats, update frequencies, and inconsistent definitions.
After hours of sifting, standardising, and cleaning, she ends up with data that feels accurate enough to use. Yet, by the time she’s ready to make recommendations, some data is already outdated, risking the firm’s ability to make timely, informed decisions.
This scenario is not imaginary. Financial firms of all sizes face similar struggles with data quality daily. Relying on multiple, often disjointed sources can leave gaps and create inconsistencies that slow operations and increase the risk of suboptimal investment choices. The complexity and volume of data needed for financial analysis today demand a level of accuracy and speed that manual efforts alone can’t keep up with.
The Anatomy of Data Chaos in Financial Services
Financial firms face data quality challenges that impact their daily operations and bottom line. These issues run deeper than simple spreadsheet errors.
Fragmented Sources
Picture a derivatives trader needing to price a complex instrument. They must pull data from Bloomberg, cross-reference it with internal risk metrics, and validate against last quarter’s reports stored in a legacy system. Each source speaks its own language. Bloomberg shows prices in decimals, while internal systems use fractions. What should be a 15-minute task becomes a two-hour reconciliation project.
Manual Processing
Analysts spend 40% of their workday copying and pasting data between systems at a large asset management firm in Boston. One misplaced decimal point in a million-dollar transaction isn’t just a typo—it’s a compliance risk and potential financial loss. Despite triple-checking their work, errors slip through during high-pressure periods.
Inconsistent Metrics
A portfolio manager in London pulls the debt-to-equity ratio for a potential investment. The research terminal shows 1.5, but the internal analytics platform displays 1.8. Both numbers are technically correct—they’re just calculated using different methodologies. These inconsistencies force teams to maintain complex documentation explaining each variation, adding another layer of complexity to their workflows.
A Day in the Life of a Financial Analyst without Automation
7:30 AM: James arrives at his desk, dreading the data consolidation ahead. His morning starts with downloading yesterday’s trading data from three different systems.
8:45 AM: He spots irregularities in the Asian market data. The currency conversions don’t align with the standard format. He emails the Singapore office for clarification.
10:30 AM: Still waiting for a response, James manually adjusts formats in Excel to match house standards. He’s already found two formula errors in linked spreadsheets.
12:05 PM: Singapore has dealt with its own busy day and responds with the corrected data. James must redo three hours of work because the initial numbers were wrong.
2:15 PM: The risk management team needs updated exposure calculations. James postpones his work to prioritise this urgent request, knowing he must work late to catch up.
This seems like a nightmare and yet is an average day for many analysts working at financial firms. They are distracted from focusing on the core business and this incurs delays in deal-making.
Is this acceptable in a world where AI can assist us in every instance? Not at all, right? Let’s see how AI-driven automation can help financial firms improve such scenarios.
Transforming Data Management in Financial Services
When James’ firm implements automated data management, his daily routine is transformed. The system handles the heavy lifting of data processing, allowing James to focus on analysis, strategy and recommending deals.
Automated Data Collection
Modern automation platforms connect directly to data sources, standardising information as it flows in. When trading data arrives from Tokyo, it’s automatically converted to the required format and cross-referenced against other sources. What once took hours now happens in seconds.
Data Cleaning and Validation
Smart validation immediately flags anomalies. When a trading partner submits unexpected data, the AI system identifies the issue and corrects it based on its training and experience. This prevents bad data from contaminating downstream settlement.
Real-Time Updates
Teams now work with live data instead of waiting for end-of-day reconciliations. When market conditions change, portfolio managers see the impact on their positions in real-time, allowing for faster, more informed decisions.
Consistency and Return on Investment
Automated data management makes a difference at any financial firm and delivers these outcomes:
- Reduction in report preparation time from several days to a few minutes
- Improvements in data accuracy rates from 92% to 99.7%
- Analysts will be able to spend 80% of their time on strategic analysis instead of data cleanup
- The firm can save over $250,000 annually by eliminating redundant data validation roles and saving time for their analysts.
The benefits and falling costs of automating data preparation make investing in AI workflows a no-brainer for any financial services firm. Where do you start and how do you ensure successful adoption of automation?
Practical Steps to Adopt Data Automation in Your Firm
- Start with an audit of your current data flows. Identify where manual intervention causes the most delays and errors.
- Choose Your Battles. Begin with one critical process—perhaps daily NAV calculations or risk reporting. Success in one area builds momentum for broader adoption.
- Build Internal Support. Document the hours spent on manual data processing. Show stakeholders how automation will free up resources for higher-value activities.
- Select the Right Tools. Look for solutions that:
- Integrate with your existing systems
- Offer strong validation capabilities
- Provide clear audit trails
- Scale with your business.
- Implement in Phases Start with a pilot program in one department. Use the lessons learned to refine your approach before expanding.
At the start, it might seem overwhelming to adopt AI automation in your firm. Thus, it’s advisable to partner with companies like MSBC Group, which have the industry understanding and expertise in building multi-agent workflows and systems.
The path to automated data management isn’t about replacing human judgement—it’s about empowering financial professionals to spend more time applying their expertise to complex problems instead of wrestling with spreadsheets.
Get in touch with us today to initiate a discussion on how we can help you automate and scale easily.